AI-infused 3D modeling of substations, pump houses, and other facilities offers utilities immediate benefit
By Ben Dwinal
Using advanced GIS systems, utilities can create detailed infrastructure models that enable them to deliver services to residents more efficiently and safely. These models include highly granular data about the networks of lines and pipes that deliver electricity, water, and natural gas to customers.
Far too often, these models are missing a critical category of data: detailed information about facilities that are key junctions in their networks, including substations, vaults, pump houses, regulator stations, pipeline junction boxes, and other locations with a dense distribution of assets.
Blind Spots in the Network
For many utilities, these facilities are blind spots for their GIS systems. In engineering terms, they are “black boxes” where you can see what goes in and what comes out, but you have little to no information about what is inside of them. With black boxes like this, utilities face a long list of questions that create challenges, headaches, and operational inefficiencies.
What equipment is there? How old is it? Who manufactured the equipment? Are there any recalls or maintenance concerns about that equipment? What are the most likely points of failure? What will the work crew need in their truck to successfully carry out a work order? Without these assets accurately mapped and present in the GIS system, organizations reduce the analytical and visualization capabilities of modern geospatial solutions.
For many utilities, the only way to find out what is in these black boxes is to view a tabular list in the asset system or roll a truck with a crew to manually gather information about each piece of equipment in these facilities, including make, model, serial number, pressure or voltage of throughput, and X, Y, Z location. These truck rolls are a slow, inefficient process that brings workflows to a halt and prevents teams from focusing on more valuable tasks.
If any work needs to be done on the site, a second truck roll is required. The first is purely reconnaissance to see what is there. If you multiply this by the massive number of these types of facilities for a given utility, that is an enormous amount of inefficiency. Reducing return site visits can save significant operating costs for most utility organizations.
Fully Realizing the ROI of GIS Investments
Even if truck rolls allow the resolution of a specific task, that information does little to improve the data that their GIS systems rely upon. Manually collected information typically falls far short of the accuracy needed by geospatial systems.
Capturing traditional photos of these facilities also falls short of that threshold because photos are too inconsistent, incomplete, and low quality to extract key details. Technology like lidar could hypothetically be used to automate this data collection, but it is still too labor intensive and expensive to be efficient.
Manual reconnaissance on an ad hoc basis is the best available solution for allowing work orders to move forward, but that does nothing to solve the overall issue: the black boxes that exist throughout utilities’ digital infrastructure networks. These blind spots stand in the way of utilities achieving the full ROI of their investments in advanced geospatial systems.
As an example, this data is critical for fully realizing the enormous benefits of migrating to the Esri Utility Network (UN). Esri’s UN allows you to connect transmission and distribution in the same network model, but it requires timely and accurate correction or creation of facility data to achieve the highest ROI. Without it, facility visits take longer, projects suffer, and overall field team effectiveness decreases.
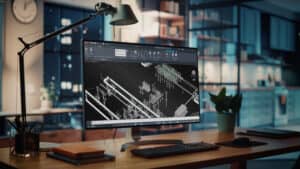
Scanning utility substations and placing the information in GIS tools can save utilities costly manpower.
Shining an AI-Driven Light into the Dark
The days are numbered for these black boxes, though. New technologies are giving utilities a fast, accurate way to convert those blind spots into granular, geospatially accurate information about the equipment they contain.
Using specialized 3D cameras and AI-driven machine learning (ML) modeling that uses computer vision, utilities can now collect high-quality facilities’ data in a highly automated way. With a single visit, image capture teams can record a location’s complete collection of equipment and its geospatially precise location. From there, image processing tools identify specific equipment and extract key details like their serial numbers.
The first step involves capturing the imagery data by walking through the facility using 360-degree camera technology. This type of camera secures a visual representation of the interior using 3D point clouds. Once captured, the next step is for this imagery to be fed into ML models that can find and label each piece of equipment, including valves, meters, regulators, pipes, as well as electric equipment. Visually legible serial numbers and other data are also extracted and stored with the new feature record and the z-enabled spatial location.
To illustrate this in action, let’s look at one gas regulator station in a utility’s network. For most utilities today, this facility will be represented in a GIS database as a single data point with no additional information. If you were to manually collect information about the contents of that regulator station and enter it into the utility’s GIS system so that it could be linked to the asset management system, the process might take 10 hours of labor, which is a massive time commitment for a single, small asset.
When you consider that utilities may have thousands or tens of thousands of these stations, the human power needed for this process is simply not practical. In contrast, high-resolution, terrestrial image capture and AI-enabled ML modeling can complete the same process in mere minutes. A process that might take months or years using traditional methods could be completed in just days for all gas regulator stations in a network.
This 3D terrestrial imaging and object detection quickly and efficiently delivers a complete, accurate asset record of the equipment in these locations, eliminating blind spots in utility’s GIS systems. This unlocks capabilities in advanced GIS systems like Esri’s UN, including the ability to remotely inspect a facility, identify needed maintenance tasks, and prepare for new maintenance and construction projects without extra truck rolls.
This brings AI-driven automation to a huge source of inefficiencies for utilities, saving time, lowering costs, and replacing manual, tedious work activities so dedicated staff can perform higher-value work. The data derived from this 3D modeling of stations is also a powerful source of new data-driven insights that utilities can derive from their GIS, ERP, WMS, EAM (Enterprise Asset Management), and other enterprise systems.
Removing these black boxes and replacing them with critical data about these blind spots in networks is a game changer for utilities that immediately delivers benefits not only in the short term but for the future of their operations.
Ben Dwinal is the vice president of Solution Architecture at Locana, a TRC Company. Locana is a location and mapping technology company whose software products and services solve the world’s most pressing infrastructure, sustainability, business, and social challenges.